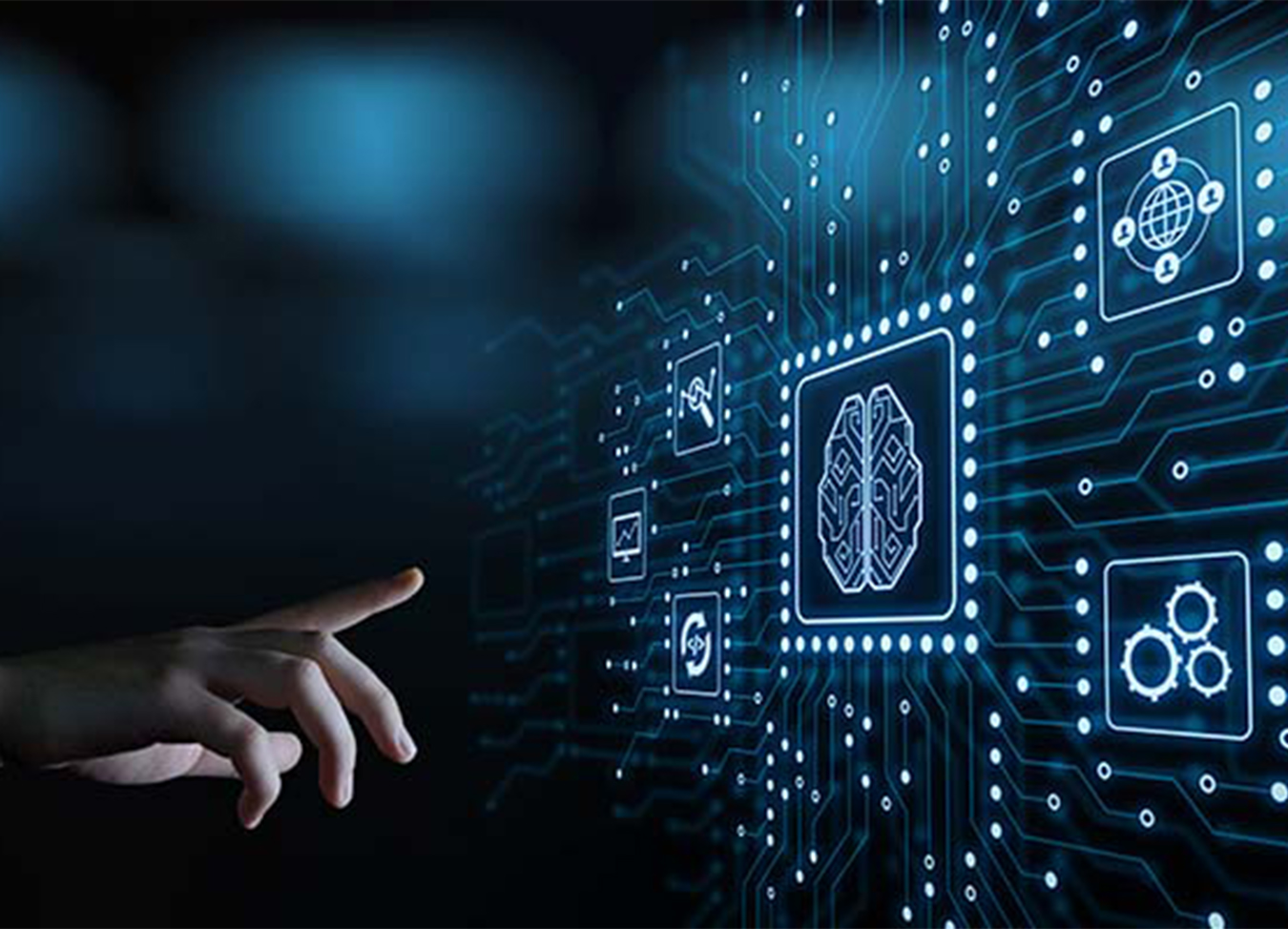
Machine learning has been around for more than 70 years and has come a long way since the late 1950s when Frank Rosenblatt paved the way with his design for the first artificial neural network that implemented patterns and shapes recognition. And while machine learning hasn’t been around quite as long in the oil and gas industry, its applicability and use is growing. We know for certain that price volatility in this industry continues to be a challenge, and this is where machine learning models can help.
In the early 2000s, when things got tough for the Oakland A’s, Billy Beane turned to machine learning to change the game of baseball. Early machine learning methods were used to analyze on-base and slugging percentages to identify anomalies, uncovering players with potential that traditional scouting methods would have overlooked. Similarly, with machine learning, pricing teams can gain insights and react faster than traditional methods. Let’s look at how it works.
The Science Behind Machine Learning: Patterns and Predictions
As a former pricing manager, I know firsthand how margin leakage and volume performance can keep someone up at night and how easy it is to get tangled up in the data. Years of pricing history is typically captured in spreadsheets and databases, with pricing managers often lacking the time and tools to effectively mine and model the data. Together with constant market volatility and competitive pressures, it becomes increasingly harder to spend time optimizing pricing strategies.
When using historical knowledge and data to predict the future, the human mind can only absorb and process so much. Machine learning allows for speed and scale by augmenting the intimate knowledge that typically resides with only a few people. It can be the vehicle to uncover margin opportunities, and given the scale of volumes in our business, seemingly small gains in unit margin can result in significant performance improvement.
Like how Billy Beane used machine learning models to uncover small performance differentiators for players that added up a winning team, machine learning models are helping pricing managers improve sales performance and arrive at the optimal price.
Using Pricing Tools to Arrive at the Optimal Price
Pricing teams can use the predictive power of machine learning algorithms, taking action today based on analysis of what happened in the past. Without it, they rely primarily on organizational memory or their team’s knowledge about what happened in previous periods and manually run comparisons.
In 2020, we all experienced the most unpredictable year in recent history. Recalling what volume might have looked like for Labor Day 2020 and pricing similarly this year would not be the best approach. Still, if you could compare and model what it looked like over the last three years, your forecast accuracy for this year would improve because of your ability to analyze the differences and uncover patterns. Or you might want to look at similar holiday weekends in the current year.
Machine learning provides the insights to make more informed pricing decisions which can give you a strategic advantage. Let’s explore some machine learning use cases.
How Advanced Models Can Improve Volume and Margin Growth
A typical use case for machine learning is to predict volume and margin values for a range of prices. We tested some machine learning models when trying to solve for increasing volume and making the maximum profit based on volume target. Then we built an optimization process to achieve that target volume. The parameters and results of this approach vary based on customer behavior and market conditions.
It isn’t easy to achieve a volume growth strategy using manual processes because you can’t react quickly. You are either setting the price early and leaving margin on the table or not early enough to achieve your target volume. With machine learning models, you can find the sweet spot for each part of the day and better react to changes to achieve your target volume goals.
The strength of these models is that they give you insights into individual customer behavior. In one illustrative example, a customer had a higher probability of lifting a second time than was expected. The customer ordered in the morning, so the expectation was that they would be less likely to lift later in the day. After further analysis, it was evident that the customer in question behaved like this in the real world. The model illustrated how certain customers were three times more likely to lift if they had already lifted earlier that day. This underscores an opportunity to capture additional margin by understanding individual customer behavior.
Reaching Your Full Pricing Potential
If you’re not leveraging digital pricing platforms today to undertake one of the most critical activities in this industry, you risk falling behind. Relying on institutional knowledge about past performance rather than capturing historical data and leveraging machine learning is simply not sustainable or scalable.
Today, machine learning can be a game-changer in the wholesale fuel industry giving pricing managers more time to focus on their strategies to deliver incremental value rather than the arduous pricing process itself. Using one system to price every segment of your business can give you greater control and flexibility.